Valo
Breakthroughs in health could be engineered?
New biological and chemical experimental platforms and the application of computing to human data can shave years off drug development and improve the likelihood of success by finding previously hidden associations between biomarkers for disease, drug targets, and therapeutic molecules.
In the pharmaceutical business, failure is the rule. Historically, only one in 40 hypotheses about molecules that might interrupt a disease process leads to the identification of a drug candidate. Only one in 20 of these candidates makes it all the way through the three phases of clinical trials and wins FDA approval. And only one in five drugs that reach the market earns a positive return. Do the math. “We’re in an industry where one in 4,000 is considered successful,” says Flagship Pioneering general partner David Berry. “The bleeding edge wants to make this two or three in 4,000. That’s just not good enough. Our health and lives are at stake.”
Flagship Pioneering founded Valo in early 2019 to change how drugs are developed. Valo engineers advances that lead to high-impact breakthroughs by applying emerging techniques in computing to human data, reducing the cost and time of drug development while increasing confidence the medicines will work. Berry, Valo’s CEO, who co-founded more than 30 companies at Flagship, including Valo, says, “It turns out that an innovative company can come in, construct the right sort of data foundation from scratch, apply infinite-scale computing, and effectively break free of historical dogma about how drugs are developed.”
Valo has created a unique closed-loop system that allows for proprietary drug candidates to be created with greater efficiency and precision. The system couples computationally driven and empirical approaches, yielding a wealth of powerful drug candidates, including molecules aimed at hard-to-drug and so-called undruggable targets. Finally, Valo is using new computational techniques to bring more predictive confidence to clinical trial design and execution.
Valo’s initial focus is on treatments for cardiovascular diseases, neurodegeneration, and cancer. Its suite of computational tools—collectively known as Opal—is designed to identify new drug candidates in each disease area; evaluate new and known molecules to forecast how they’ll interact with protein targets in the body; predict which patients will benefit most; and design increasingly efficient clinical trials. “The only way to have a transformative effect on this industry is to do it soup to nuts,” says Valo’s head of data science and artificial intelligence, Brandon Allgood, who leads development of the company’s technology platform. Already, he says, Opal has uncovered potential new ways to treat Parkinson’s disease, cardiovascular disease, and glioblastomas, the deadliest type of brain tumor, among other diseases. Valo seeks to have 30 preclinical programs and six clinical programs under way within three years, says Hilary Malone, who left a position as Sanofi’s chief regulatory officer to become the startup’s chief operations officer, pharmaceuticals. Industry insiders know that “the traditional drug discovery and development paradigm is broken,” Malone says. Large drug companies are attempting to digitize the clinical trial data they use to meet regulatory requirements but are unable to tackle the larger transformations needed to take advantage of the capabilities of today’s AI. “To be able to embed a compute-centric approach in the heart of a decades-old human-centric process is the type of profound transformation that traditional pharma companies cannot possibly make,” she says.
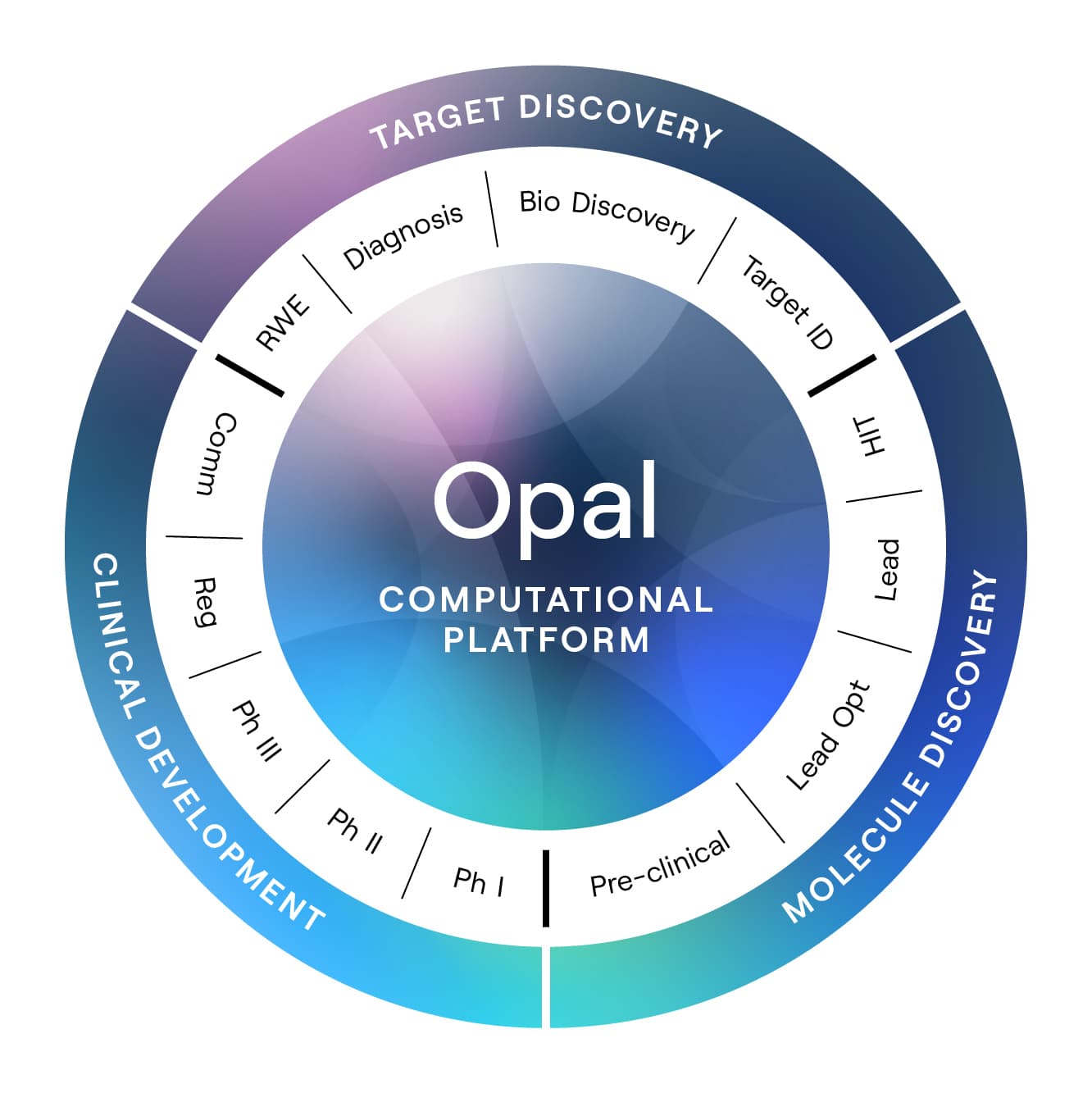
Valo was built to solve that problem, in part by giving biologists, chemists, data scientists, computer scientists, and drug developers the space to bridge their disparate languages and cultures. A simple example, Allgood points out, is the term “API.” To software developers it means one thing (application programming interface) and to drug researchers it means something completely different (active pharmaceutical ingredient).
That makes company meetings interesting. But it also means “my team spends a lot of time observing the scientists and understanding how they think about a problem,” says Allgood, who joined Valo when it acquired Numerate, a computational chemistry startup he co-founded. “The mantra that encapsulates that is, ‘Encode the physics you know and let the machine learning do the rest’”—not in order to cut scientists out of the loop but in order to help them test more hypotheses, faster. “We’re trying to figure out how to leverage the best of human intelligence and machine intelligence,” Allgood says.
The output of a machine learning algorithm, however, is only as good as the data it has to work with. Therefore, Valo has assembled a unique data lake that includes multiple agreements with national healthcare systems, as well as exclusive data about diseases. The data follows patients across decades as they develop symptoms and respond to various treatments. But Valo has also matched up healthcare records with patients’ genomes, proteomes, metabolomes, microbiomes, and even radiographic images and pathology samples. It’s “one of the richest, biggest, most pristine clinical data sets in the world,” Allgood says.
One use of this data will be to help Valo sidestep a frequent challenge in drug development: clinical trials that end in disappointment because the drugs weren’t tested on the right populations. “One of the things we’re doing is identifying patient subpopulations, looking at the biomarkers associated with that subpopulation, understanding how those biomarkers relate to the underlying biology and targets, and looking for a genotype identification,” Allgood explains. “So by the time we have a target and we enter discovery, we already know what responder population we’re going to use in Phase 2 clinical trials, because that’s how we got to that target.”
One big advantage that Valo has, according to Malone, is that it was founded at a time when three important trends had converged. “Number one is access to data that was not previously there. Number two is the computational power of the cloud. And number three is that for medicine, the low-hanging fruit has been picked. The easy pathways have been elucidated. The true unmet medical need going forward is to solve the problems that the human brain alone cannot solve.” Malone notes that the drug industry has spent billions of dollars researching new treatments for Alzheimer’s disease over the last decade, with almost zero success. “But it has generated a ton of data. It’s only through applying the capabilities of human and machine learning that we’ll actually solve these complex problems.”
In David Berry’s eyes, Valo is contributing to a new definition of disease, one based not on clusters of symptoms but on underlying biological mechanisms and the way they emerge and shift in individuals over decades. To explain the difference, he reaches for an analogy from weather forecasting. “If you look across a few hundred thousand patients and the common paths of their disease, it’s almost like the spaghetti plots of all the different ways a hurricane might go.” Designing drugs to intervene in disease is like forming a plan to get people out of the path of their own medical hurricane—except that “now you can actually start from the right foundation.”
If Valo has placed its bets correctly, Berry says, “I think we’ll massively cut costs. I think we’ll massively cut time. And I think we’ll be able to bring forth treatments to diseases which have previously been deemed untreatable.” And that could change the odds in everyone’s favor—and also change medicine as we know it.
Latest News from Valo
- Charles River and Flagship’s Pioneering Medicines Enter Agreement Leveraging AI-Powered Logica with Aim of Creating Novel Therapies for Unmet Medical Needs 02.16.2023
- Charles River and Valo Launch Logica, an Integrated AI-Powered Drug Discovery Solution to Rapidly Deliver Optimized Preclinical Assets 04.27.2022
- Valo Health Acquires TARA Biosystems Creating First of its Kind Vertically Integrated Cardiovascular Platform 04.05.2022
- Charles River and Valo Health Announce Strategic Partnership to Provide Clients with Access to AI-Enabled Drug Discovery Solutions 01.19.2022
- Flagship Pioneering Announces Valo Health to Transform Drug Development 09.23.2020